

Hanoona Rasheed
Ph.D. Student in Computer Vision (MBZUAI - IVAL LAB)
I am a Ph.D. student in Computer Vision at MBZUAI, working under the supervision of Dr. Salman Khan and Dr. Fahad Khan at the IVAL lab.
My current area of research is focused on exploring the potential of multi-modal understanding from vision and language to build scalable general-purpose vision systems, that continually learn and can generalize to various domains and downstream tasks using an open-vocabulary.
Prior to joining MBZUAI, I worked as a Signal Processing Engineer in Chemometrics R&D at Robert Bosch.
Publications
*Hanoona Rasheed, *Muhammad Maaz, Sahal Shaji, Abdelrahman Shaker, Salman Khan, Hisham Cholakkal, Rao M. Anwer, Eric Xing, Ming-Hsuan Yang, Fahad S. Khan
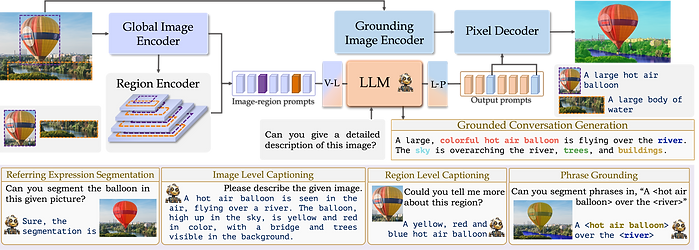
Grounding Large Multimodal Model (GLaMM) is an end-to-end trained LMM which provides visual grounding capabilities with the flexibility to process both image and region inputs. This enables the new unified task of Grounded Conversation Generation that combines phrase grounding, referring expression segmentation and vision-language conversations. Equipped with the capability for detailed region understanding, pixel-level groundings, and conversational abilities, GLaMM offers a versatile capability to interact with visual inputs provided by the user at multiple granularity levels (objects, object parts, attributes, relationships and holistic scene understanding).
*Muhammad Maaz, *Hanoona Rasheed, Salman Khan, Fahad Khan

Video-ChatGPT is a video conversation model capable of generating meaningful conversation about videos. It combines the capabilities of LLMs with a pretrained visual encoder adapted for spatiotemporal video representation.
*Hanoona Rasheed, *Muhammad Uzair Khattak, Muhammad Maaz, Salman Khan, Fahad Khan

In this work, we formulate and show the significance of an often neglected but simple baseline for transferring image-based CLIP model to video domain. ViFi-CLIP (Video Fine-tuned CLIP) shows that a simple fine-tuning of CLIP is sufficient to learn suitable video-specific inductive biases, and can perform competitive to more complex approaches having dedicated components designed to model temporal information in videos. We introduce base-to-novel generalization benchmark for video-domain for evaluating the generalization ability of models for video action recognition.
Muhammad Uzair Khattak, Hanoona Rasheed, Muhammad Maaz, Salman Khan, Fahad Khan

In this work, we propose to learn prompts in both vision and language branches of pretrained CLIP for adapting it to different downstream tasks. Previous works only use prompting in either language or vision branch. We note that using prompting to adapt representations in a single branch of CLIP (language or vision) is sub-optimal since it does not allow the flexibility to dynamically adjust both representation spaces on a downstream task. To this end, we propose Multi-modal Prompt Learning (MaPLe) for both vision and language branches to improve alignment between the vision and language representations. Our design promotes strong coupling between the vision-language prompts to ensure mutual synergy and discourages learning independent uni-modal solutions.
*Hanoona Rasheed, *Muhammad Maaz, Muhammad Uzair Khattak, Salman Khan, Fahad Khan


In this work, we propose to solve the Open-vocabulary detection (OVD) problem using pretrained CLIP model, adapting it for object-centric local regions using region-based distillation and image-level weak supervision. Specifically, we propose to utilize high-quality class-agnostic and class-specific object proposals via the pretrained mulit-modal vision transformers (MViT). The class-agnostic proposals are used to distill region-specific information from CLIP and class-specific proposals allows us to visually ground large vocabularies. We also introduce a region-conditioned weight transfer method to get complementary benefits from both region-based distillation and image-level supervision.
*Muhammad Maaz, *Hanoona Rasheed, Salman Khan, Fahad Khan, Rao M.Anwer, Ming-Hsuan Yang

In this work, we explore the potential of the recent Multi-modal Vision Transformers (MViTs) for class-agnostic object detection. Our extensive experiments across various domains and novel objects show the state-of-the-art performance of MViTs to localize generic objects in images. We also develop an efficient and flexible MViT architecture using multi-scale feature processing and deformable self-attention that can adaptively generate proposals given a specific language query.
Abdelrahman Shaker, Muhammad Maaz, Hanoona Rasheed, Salman Khan, Ming-Hsuan Yang, Fahad Shahbaz Khan

The work proposes a 3D medical image segmentation approach, named UNETR++, that offers both high-quality segmentation masks as well as efficiency in terms of parameters and compute cost. Our extensive evaluations on three benchmarks, Synapse, BTCV and ACDC, reveal the effectiveness of the proposed contributions in terms of both efficiency and accuracy.
Abdelrahman Shaker, Muhammad Maaz, Hanoona Rasheed, Salman Khan, Ming-Hsuan Yang, Fahad Shahbaz Khan

The work proposes a 3D medical image segmentation approach, named UNETR++, that offers both high-quality segmentation masks as well as efficiency in terms of parameters and compute cost. Our extensive evaluations on three benchmarks, Synapse, BTCV and ACDC, reveal the effectiveness of the proposed contributions in terms of both efficiency and accuracy.
*Equal Contribution